Enabling Machine Learning: How to Optimize Infrastructure, Tools and Teams for ML Workflows
Yann Lemonnier
In this talk, we will explore the role of a machine learning enabler engineer in facilitating the development and deployment of machine learning models. We will discuss best practices for optimizing infrastructure and tools to streamline the machine learning workflow, reduce time to deployment, and enable data scientists to extract insights and value from data more efficiently. We will also examine case studies and examples of successful machine learning enabler engineering projects and share practical tips and insights for anyone interested in this field.
Yann Lemonnier
Yann Lemonnier is an experienced ML/Data Engineer with a strong background in data analysis, machine learning, and time-series predictions. He is currently focusing on enabling production machine learning projects with MLOps. Yann has a Master's degree in Physics from the Université de Sherbrooke in Canada and is a certified AWS Cloud Practitioner and GCP Professional Data Engineer. Yann is passionate about using his expertise to drive business value and innovation through data-driven insights. In his previous work experiences, he has worked as a Data Engineer, Tech Lead, Machine Learning Engineer, Flight Test Data Analyst and acoustic engineer. Yann has supervised data scientists teams, designed software solutions, and did project screening and reviews. Some of his past projects include L’Oréal supply chain ETL, tabular data AutoML for Aircraft design offices, Machine activity monitoring from vibration IoT, Aircraft Noise Classification, Aircraft Vibration Event Impact Assessment, Aircraft External Noise Cartography, and vibration flight test analysis. Yann has worked in companies like L’Oréal, Alteia, COREIoT, and Airbus Flight Test Center. He is now working at Adevinta in the ReCommerce (second hand marketplace) industry.
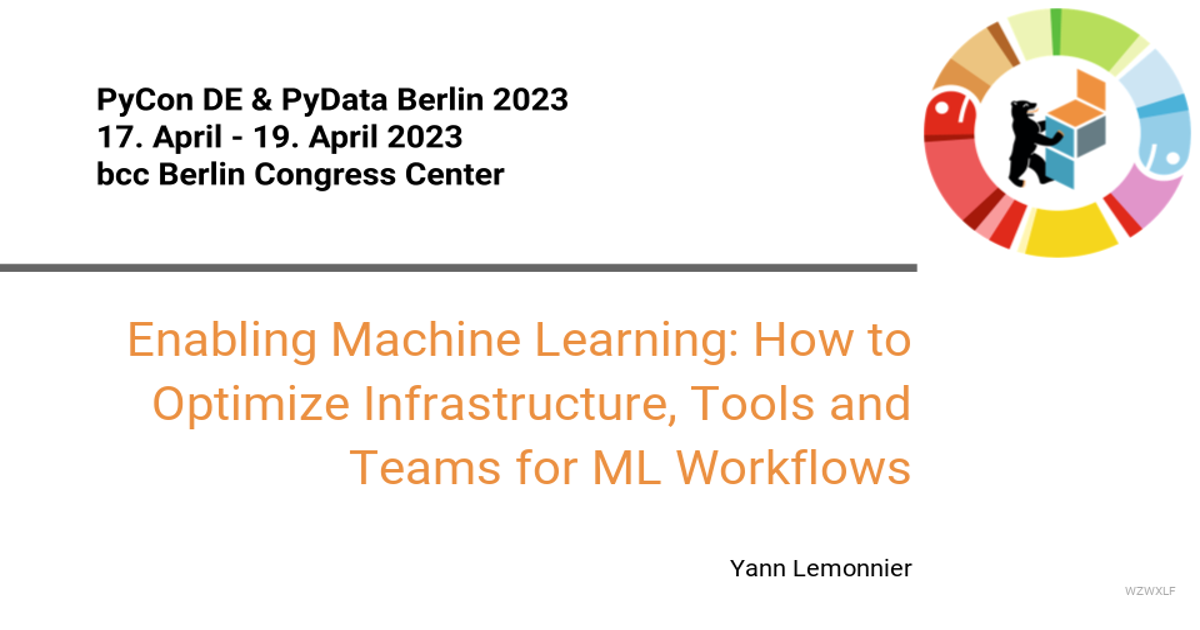