BHAD: Explainable unsupervised anomaly detection using Bayesian histograms
Alexander Vosseler
The detection of outliers or anomalous data patterns is one of the most prominent machine learning use cases in industrial applications. I present a Bayesian histogram anomaly detector (BHAD), where the number of bins is treated as an additional unknown model parameter with an assigned prior distribution. BHAD scales linearly with the sample size and enables a straightforward explanation of individual scores, which makes it very suitable for industrial applications when model interpretability is crucial. I study the predictive performance of the proposed BHAD algorithm with various SoA anomaly detection approaches using simulated data and also using popular benchmark datasets for outlier detection. The reported results indicate that BHAD has very competitive predictive accuracy compared to other more complex and computationally more expensive algorithms, while being explainable and fast.
Alexander Vosseler
Affiliation: Allianz Germany - Advanced Analytics Claims
Alexander Vosseler works as Principal Data Scientist at the Advanced Analytics Claims team of Allianz Germany - Chief Data Office in Munich. He has many years of industry experience as a data scientist and holds a PhD in Statistics with majors in Bayesian and Computational statistics. During his industry career as a data scientist he worked for companies such as Siemens AG, Allianz Global Corporate & Specialty SE and Allianz Germany.
His current methodological interests lies in probabilistic machine learning and uncertainty quantification with applications in time series methods, anomaly detection and NLP. In his spare time he likes to go jogging and play the drums.
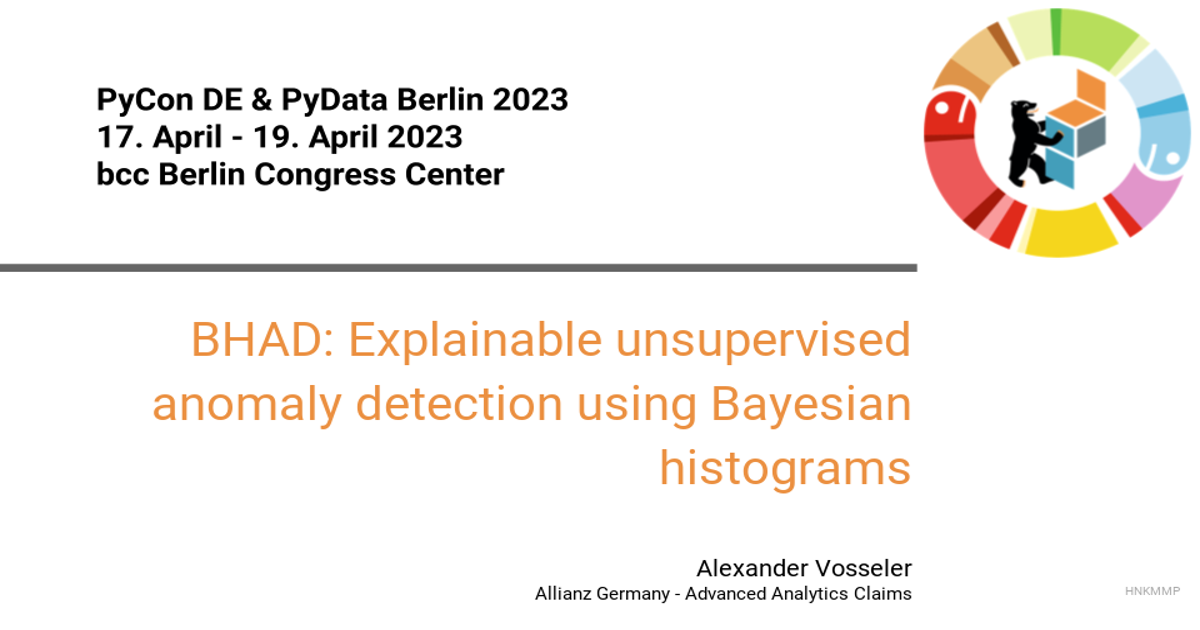