Grokking Anchors: Uncovering What a Machine-Learning Model Relies On
KIlian Kluge
Assessing the robustness of models is an essential step in developing machine-learning systems. To determine if a model is sound, it often helps to know which and how many input features its output hinges on. This talk introduces the fundamentals of “anchor” explanations that aim to provide that information.
KIlian Kluge
Affiliation: Inlinity
My journey into Python started in a physics research lab, where I discovered the merits of loose coupling and adherence to standards the hard way. I like automated testing, concise documentation, and hunting complex bugs.
I completed a PhD on the design of human-AI interactions and now work to use Explainable AI to open up new areas of application for AI systems.
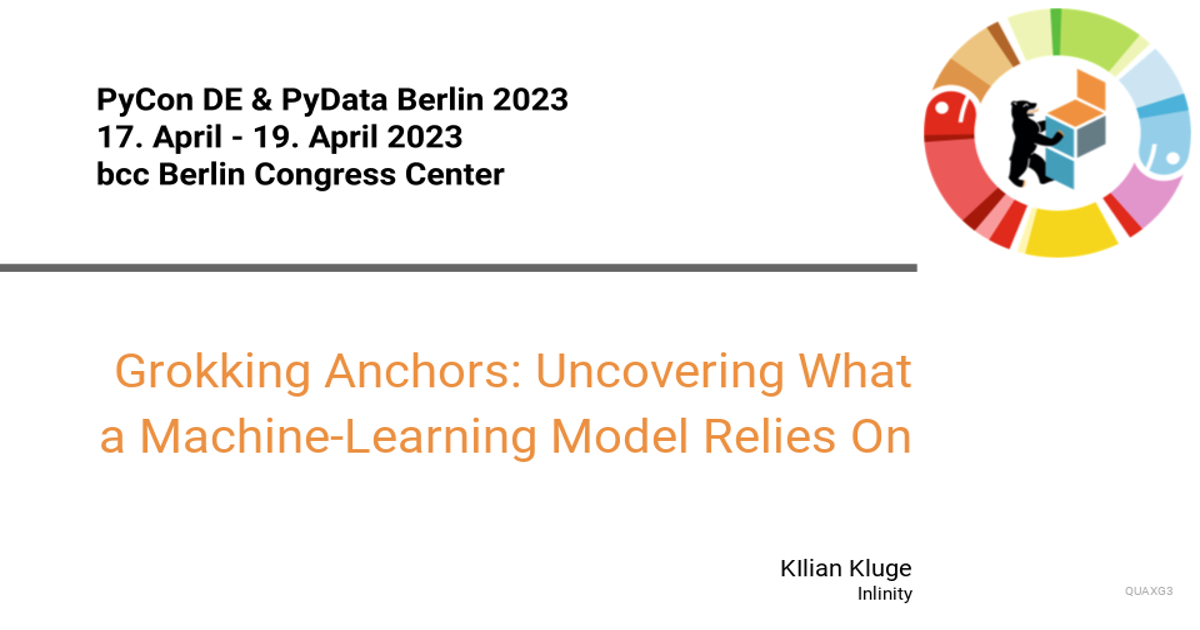