Staying Alert: How to Implement Continuous Testing for Machine Learning Models
Emeli Dral
Proper monitoring of machine learning models in production is essential to avoid performance issues. Setting up monitoring can be easy for a single model, but it often becomes challenging at scale or when you face alert fatigue based on many metrics and dashboards.
In this talk, I will introduce the concept of test-based ML monitoring. I will explore how to prioritize metrics based on risks and model use cases, integrate checks in the prediction pipeline and standardize them across similar models and model lifecycle. I will also take an in-depth look at batch model monitoring architecture and the use of open-source tools for setup and analysis.
Emeli Dral
Affiliation: Evidently AI
Emeli Dral is a Co-founder and CTO at Evidently AI, a startup developing open-source tools to evaluate, test, and monitor the performance of machine learning models.
Earlier, she co-founded an industrial AI startup and served as the Chief Data Scientist at Yandex Data Factory. She led over 50 applied ML projects for various industries - from banking to manufacturing. Emeli is a data science lecturer at GSOM SpBU and Harbour.Space University. She is a co-author of the Machine Learning and Data Analysis curriculum at Coursera with over 100,000 students.
visit the speaker at: Homepage
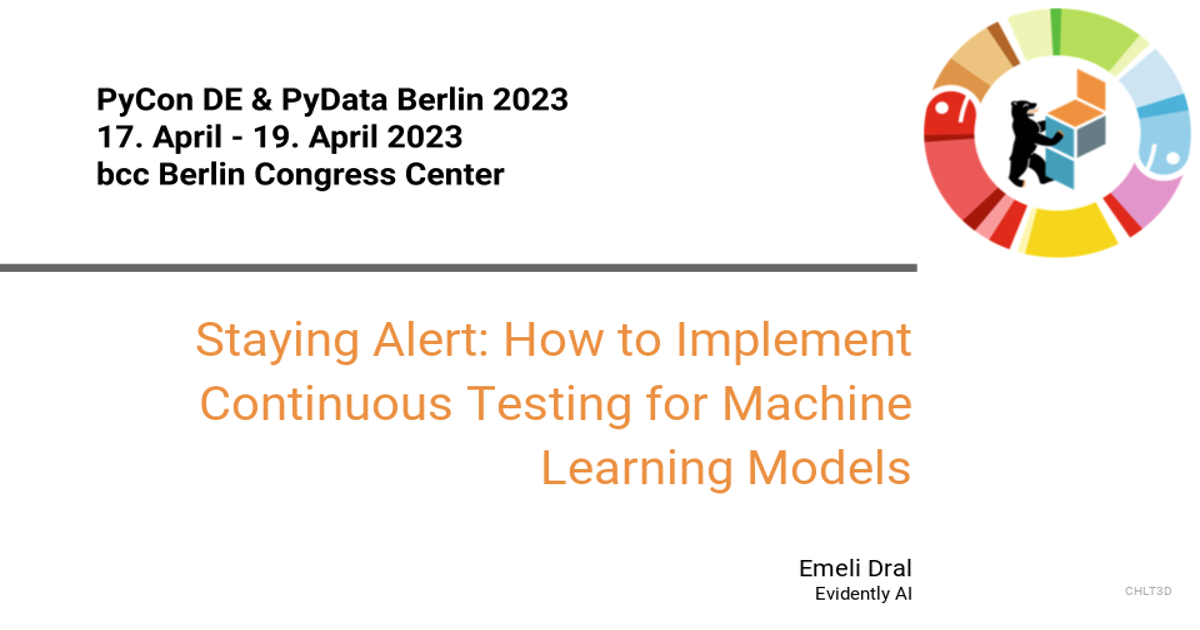